Javier Murgoitio-Esandi
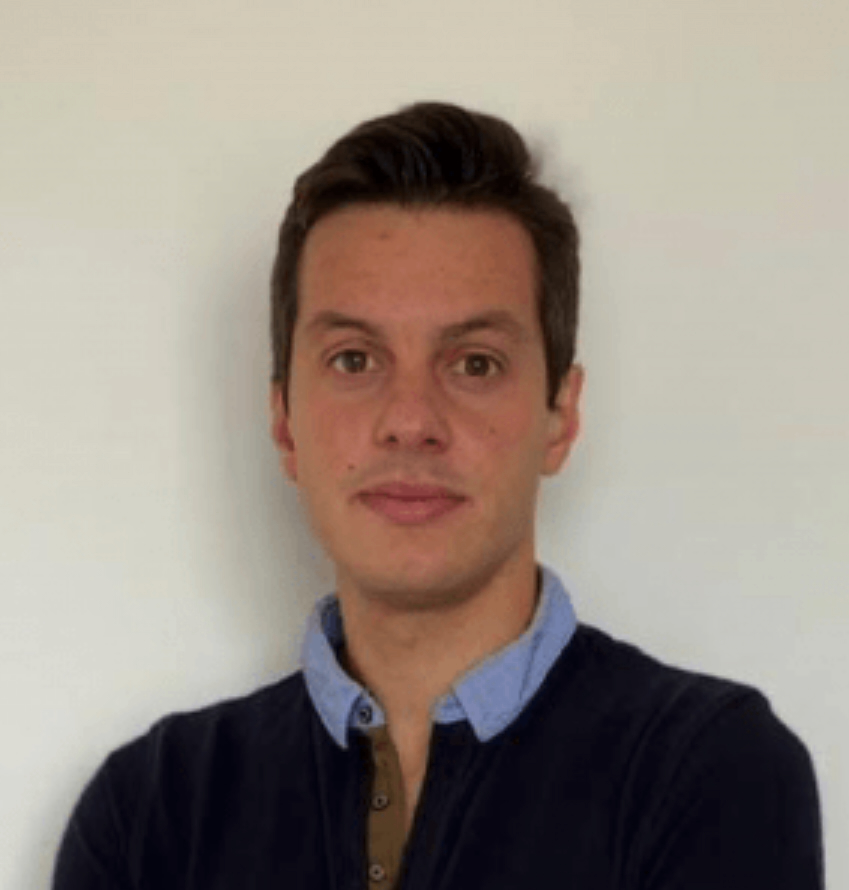
I am Ph.D student with more than 4 years of experience specializaing in the theory and application of generative deep learning methods. I am part of the Computation and Data Driven Discovery Group at the Aerospace and Mechanical Engineeirng Department of the University of Southern California, where I started my Ph.D. studies in the Fall of 2020 as part of Professor Assad Oberai’s research group. During the 2023-2024 academic year, I worked as an intern researcher at Sandia National Labs conducting research on uncertainty quantification in deep learning.
My research focuses on developing generative deep learning methods applied to anomaly detection and uncertainty quantification in medical imaging. My work on anomaly detection includes the study and development of methods such as autoencoders and energy-based models to identify rare biological events in immunofluorescent liquid biopsy images, with the end goal of aiding cancer diagnosis. As part of this project, I have also developed a foundation model for immunofluorescent microscopy images. Additionally, my research on uncertainty quantification involves the development and application of techniques such as generative adversarial networks and diffusion models to solve inverse problems in the context of elastography imaging.
As a consequence of my research and studies I am expert in Generative AI, and have extensive knowledge on foundation models and large language models.
selected publications
-
- CMAMESolution of physics-based inverse problems using conditional generative adversarial networks with full gradient penaltyComputer Methods in Applied Mechanics and Engineering, 2023
- CMAMEConditional score-based diffusion models for solving inverse elasticity problemsComputer Methods in Applied Mechanics and Engineering, 2025